Merry Merry Month of AI May
This post, AI for Mortals #5, was meant to explore AI training data, but a couple funny things happened along the way.
First, diving into that topic rearranged how I think about myself as a creator, and caused me to do a bunch of research and technical work that didn't directly make words for you. Why? That's an interesting story I'm eager to share...next time! That's when we really are going to tackle training data, and that's where it belongs.
Second, my wife and I were traveling for about half of May, which kept me mostly away from the news, even the AI news. On our return, I plowed into the backlog of new developments, and what a surreal, mind-blowing experience! If you've been with AI for Mortals for awhile, you know we're not primarily about tech industry news or "inside baseball". But I owe you a post already, and this pace really does demand notice.
So let's take a look at the incredible news from a single month of the new AI. (For the record, this is not the new AI for Mortals normal. Starting next time, we'll be back to looking at broad AI themes and what they mean for us as citizens and human beings.)
What did I miss?
Of course AI is an incredibly active space, with many, many newsworthy developments every day. But if we stick to only the biggest stories, major milestones for the key players, our societies, and even the human species, just within this single month, then...uh...we still can't come close to mentioning all of those. But let's try!
Big Tech makes big moves
Changing of the guard department: NVIDIA, the dominant maker of AI chips, momentarily blew by Apple Computer to become the world's second-biggest company by market value, behind only Microsoft. (Yes, largest in the world, not just the US, the title below notwithstanding...)
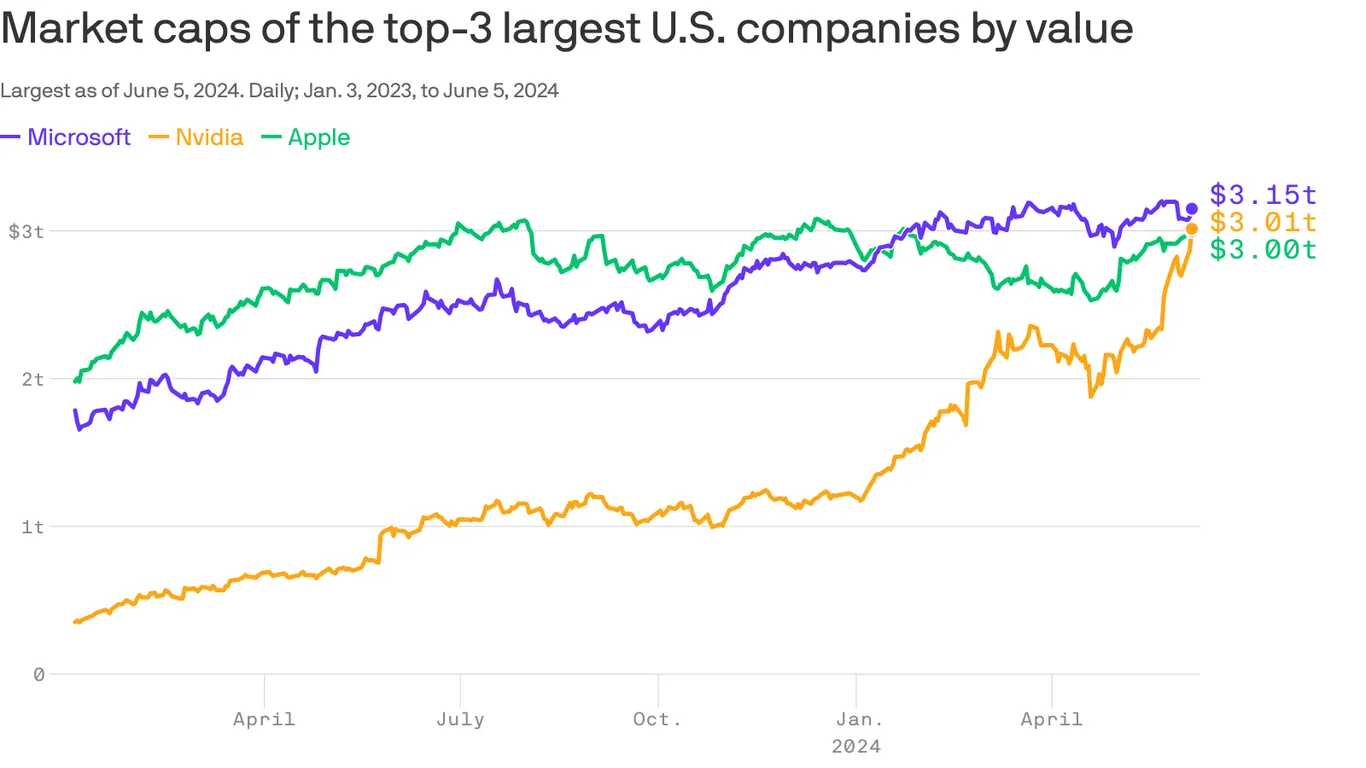
Since then, Apple has joined the AI fray in a serious way by presenting Apple Intelligence at its 2024 Worldwide Developer Conference, which is ongoing as I write. So far the market loves Apple Intelligence, and Microsoft has already been on an AI-fueled tear, so those companies are back at positions 2 and 1 respectively. But NVIDIA isn't far behind, and its recent climb has been much steeper. So we shall see.
Oh, and by the way? On June 14, NVIDIA launched Nemotron-4, an LLM family of their own said to perform on par with the original GPT-4. They say they're targeting it to the specific use case of giving "developers a free, scalable way to generate synthetic data that can help build powerful LLMs". This is huge, and raises many questions, but it wasn't May, so on we go...
OpenAI released the astonishing new models GPT-4o and ChatGPT-4o (the os are lower-case letters), and got into a fight with Scarlett Johansson. More on that below. The company also said it has begun training GPT-5 for release by the end of this year, suffered multiple major defections and whistle blows, disbanded its best-known safety team, was caught in an outrageous attempt to legally muzzle exiting employees, and reaffirmed its alleged commitments to safety and responsibility. Its efforts to sign content deals with publishers seemed to be gaining traction, even as it continued to face lawsuits from The New York Times and others.
On May 8, Google's DeepMind unit announced AlphaFold 3, a molecular structure prediction system that's more than a breakthrough; it's a breakthrough factory. I truly believe people centuries from now may look back on this moment as one of the great turning points in scientific and medical history. As its builders say,
Our AI system is helping to solve crucial problems like treatments for disease or breaking down single-use plastics. One day, it might even help unlock the mysteries of how life itself works.
So there's that.
The company also launched AI Overviews, a bid to rethink the mechanics — and economics — of online search. Both the concept and execution of this move have been poorly received in many quarters, but there's no indication the strategy is likely to change.
Anthropic announced that its LLM (large language model), Claude, can now use tools, which seems to indicate an attempt to pursue agentic AI: AI that can carry out assigned tasks autonomously in the real world (mostly meaning the real digital world...for now). There's historically been a tension between agentic AI and safety; since Anthropic is generally perceived to be taking safety more seriously than its competitors, it will be interesting to watch as they try to thread this needle. Also this month, Anthropic reported a major breakthrough in model interpretability — more on that below.
Microsoft introduced Copilot+ PCs, "a new category of Windows PCs designed for AI", as well as Recall, which records your interactions with your machine in great detail so you can converse with your own history using generative AI. The "spy on yourself" aspect of Recall doesn't appeal to everyone, but this is a genuinely intriguing attempt to find a transformative application for AI in everyday computing. Its launch, however, has been badly botched, leaving the company scrambling to respond. In other news, it looks like Microsoft is developing its own frontier model to compete head-to-head with the largest models from OpenAI, Google, and Anthropic. This isn't a complete surprise, since way back in March the company hired the CEO and acquired much of the tech and staff of Inflection, makers of the excellent Pi LLM, but it's still huge news. (For what it's worth, I kind of adore Pi and am eager to see whether Microsoft releases something that builds on its strengths.)
The New York Times broke a story claiming that Apple, after watching ChatGPT leapfrog its virtual assistant Siri, has undertaken "the tech giant’s most significant reorganization in more than a decade", and that "to catch up in the tech industry’s A.I. race, Apple has made generative A.I. a tent pole project — the company’s special, internal label that it uses to organize employees around once-in-a-decade initiatives". It's widely believed Apple has concluded a deal to use OpenAI's models to power Apple Intelligence, and that the company is in similar discussions with Google and perhaps others. (At the above-mentioned Worldwide Developers Conference, nothing has happened so far to contradict these May reports.)
Mortals getting restive (?)
Apple also contributed to something more subjective I feel about May 2024, which is that this has been a time for some to back off from seeing AI as a breath of fresh air and begin viewing it more in the light of a general disillusionment with Big Tech. Admittedly, I may be affected here by having consumed multiple weeks of news "in a gulp", but I do think there's been something of a sea change, at least for some people. Apple's role? The mood shift, if I'm right that there is one, may have been partly catalyzed by one of the worst-received ads in recent memory: an iPad Pro ad entitled "Crush!" In a typical reaction, Peter C. Baker says in The New York Times:
After a decade during which it felt as if computers were empowering human creativity, they now feel like a symbol of the forces that stand in creativity’s way and starve it of oxygen.
I urge you to read his comments in their entirety (30-day unlocked link).
In the first issue of AI for Mortals, I complained that "the journalists and brand managers who dominate the public discourse" have done us all a disservice by portraying the new AI solely as a "new dimension of the tech industry's product space". If that's what you've promised, then what you deliver has to speak to your users better than Google's AI Overviews, Microsoft's Recall, and whatever it is that Apple Crush! ad was trying to sell.
Anything else?
Yep, lots more!
In the United States, legislation requiring TikTok to be divested by its Chinese owners or be banned (first proposed by then-President Trump, who now opposes it) was signed into law by Joe Biden. This is an enormous story in itself, but especially exciting for us is that one potential bid is being organized as an explicit attempt to put TikTok under mortal control and rearchitect it to serve mortals' needs. There's a long road between today and such an outcome, but it's exciting to see someone demonstrate this kind of thinking!
India held its first nationwide election in the era of readily available deepfake technology, causing much concern around AI-fueled manipulation in a country already racked by deep polarization and provocative rhetoric. After the fact, almost all commentators seem to agree that none of the worst fears came to pass, with some going so far as to say AI was a net positive for democracy, or that it played a constructive role.
The Jeremy Coller Foundation and Tel Aviv University announced the $10,000,000 Coller-Dolittle Prize for the first team to crack two-way interspecies communication. Why now? Well, it's a response to a string of successes using LLMs to (begin to) understand non-human languages, such as this study showing that elephants address each other by name.
Okay, let's leave it there, but trust me, I could go on. Truly pivotal developments in the new AI have been coming not in a steady stream, but a tsunami.
And that's without even looking at the science!!!
I hope the above readout of one amazing month in the new AI has duly impressed you. But in a way, we still haven't done justice to the truly exceptional nature of this field's current progress. Here's the thing: the machine enabling this tsunami of change, whether you find it exhilarating or terrifying (or both), is the new AI's foundational science, and that is an infant science which is itself advancing at warp speed, even by the insane standards of computer science.
This month, like every other recent month, has seen an explosion of research aimed at all aspects of improving existing approaches and finding new ones. It would be impossible even to briefly summarize them here.
But let me try to give you a sense of how rapidly things are moving in just one critical area, and know that there are dozens of others that would have painted the same picture.
You can call this critical subproblem minimizing the cost of inference, where inference is what a chatbot (or any LLM) is doing when it's already been trained and you're using it to do some work, like answer a question or proofread a story. Or, you can describe the very same thing as reducing AI's carbon footprint.
As you can imagine, these are pressing concerns for private industry and academic researchers alike. And boy howdy, have the innovations been coming.
(Digression: This is one of several reasons the fairly popular comparison between AI and cryptocurrencies like Bitcoin makes no sense. Bitcoin is energy hungry by design, because it relies on proof of work from miners. It's built from the ground up to make sure that never becomes efficient.)
I get an email (TLDR AI) that summarizes AI news on a daily basis. Let's look at its Headlines and Research sections from the last full week in May. On Monday the 20th, 1 item out of 6 was about how to improve inference efficiency. On Tuesday, 1 out of 6; Wednesday 1 out of 6, this one concerning Microsoft's Phi-3 series, a set of "small language models" that allow dramatic cost/footprint reductions for important classes of problems. On Thursday, 2 out of 6; Friday, 1 out of 6. It's always like that.
On May 8, a team from Microsoft and Tsinghua University caused great excitement with a paper introducing YOCO, which is an alternative LLM architecture that seems to promise multiple orders of magnitude improvement in inference efficiency. (The improvement from switching from a gas to an electric car is, on average, something like half an order of magnitude.) Furthermore, YOCO can be combined with other innovations for even more impact.
One of these YOCO-compatible techniques is called quantization, and it's my personal poster child for how much low-hanging fruit there may still be in AI efficiency improvements. I'll say more about it below.
Reality check: If current rates of growth continue, the carbon footprint of computing, including the new AI, is going to be an ongoing concern regardless of how much its efficiency improves, just as it is for agriculture, transportation, and other major sectors of the economy.
How can I possibly keep up with all this?
That's a good question, and it has a simple answer: you can't. Neither can I, or anyone else. It's just not a reasonable goal. This is why AI for Mortals doesn't (usually) focus on the news of the day.
But your friend the book lover doesn't read every book that gets published, and if you're a news junkie, you still don't know every single thing that's in every department of every paper. Follow what calls to you, like your bookworm friend who doesn't care about Michael Crichton but knows every branch of the Brontë family tree.
We AI mortals, whether technically sophisticated or not, relate to AI as citizens and members of society.
Here's Helen Toner, Director of Strategy and Foundational Research Grants at Georgetown's Center for Security and Emerging Technology. But you may know her as the former member of OpenAI's board who was involved in Sam Altman's temporary ouster, and then removed after his reinstatement.
She gave a talk at TED2024 that speaks to what's required for mortals to find their footing.
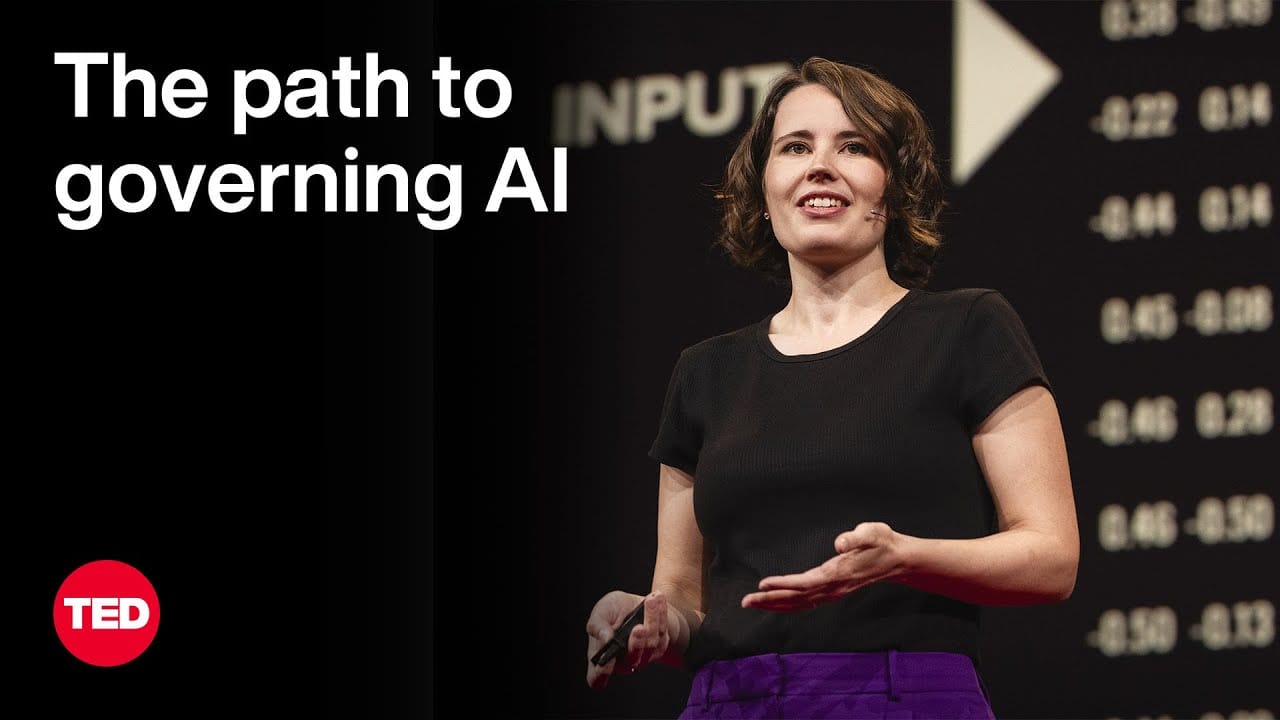
A line that sticks with me:
The way I see it, it’s not just a choice between slamming on the brakes or hitting the gas. If you're driving down a road with unexpected twists and turns, then two things that will help you a lot are having a clear view out the windshield and an excellent steering system.
Her talk is about what this means for society's relationship with AI, and I think it makes a lot of sense.
Pivotal developments
Here I'm going to say just a little more about three of the items already mentioned, to clarify why I consider each of them not merely newsworthy, but pivotal.
These aren't even the biggest things we've talked about (for that, you'd probably want to choose among AlphaFold, Microsoft's new frontier model, agentic AI, and the Dolittle prize). But almost everything covered in this post is a game changer and/or a key milestone.
Her
OpenAI's new model GPT-4o, and its incarnation in chatbot form as ChatGPT-4o, have caused a stir not because these models perform marginally better on traditional LLM benchmarks (though they do), but because they combine voice conversation, emotion recognition and synthesis, and vision with unprecedentedly low latency and smooth integration.
Basically everyone who has seen this has compared it to Joaquin Phoenix's character's interactions with the "Samantha" AI in the great 2013 (!) movie Her. (Hat tip to Luis Navarro for getting my wife and me to watch it a few years ago!) If you know only a little about ChatGPT-4o, it's probably that Scarlett Johansson, who voiced Samantha in the film, has alleged that OpenAI misappropriated her voice for "Sky", one of the ChatGPT-4o personas. This may not be true, but OpenAI has "paused" use of the Sky persona. I like "Juniper" better anyway!
I personally hear the Samantha and Sky voices as similar but not the same, especially considering that professionally-trained female speakers of the General American accent already speak within a fairly narrow envelope of constraints. But one thing I find striking: for me at least, it matters quite a bit whether I'm listening to Johansson as Samantha in Her, or to Johansson speaking in a different context. You can check this out for yourself here.
Could the incredible "that's Her!" reaction we experience with Sky have less to do with the pure sound shapes of Samantha's voice, and more to do with how fully the entire ChatGPT-4o experience recalls what we saw in the movie?
I think so. But the point is that Samantha is fiction, while Juniper — and her brothers and sisters — are real. This isn't copying. This is the fulfillment of a prophecy.
The effect is quite astonishing. Unless you've already seen (or tried!) this in action, I'd really encourage you to take a look at the demo in this OpenAI video: https://www.youtube.com/watch?v=DQacCB9tDaw. It starts at 9 minutes in. I can testify from my own experimentation that this is a fair representation of what interacting with ChatGPT-4o is like.
While we should be very impressed with what OpenAI has accomplished here, it's sobering to consider that at the moment we have exactly one secretive company (despite their Orwellian name) deciding what LLM-powered pseudo-emotional personas are to be added to the population of our world. That's too much power for one commercially-motivated gatekeeper, especially in view of "who" they chose to highlight in their product intro: the obsequious, giggly, flirty Sky.
Opening the box (a little)
On May 21, a team from Anthropic published a breakthrough paper on what's called mechanistic interpretability. In the new AI's research lingo, this means making sense of what you see when you peer inside the box of an LLM's computations.
As discussed in a previous AI for Mortals post, it's an extremely hard problem. The LLM's internal state at any stage of inference consists of a huge collection of neuron values, which depend on the many, many billions of parameters in the wall of numbers. What role any specific neuron or parameter plays is initially inscrutable. Conversely, we know from experimentation on smaller models that it's rare for anything meaningful to be tidily represented by a single neuron; instead, the meaning is smeared all over the place.
The Anthropic team attacked this "smearing" problem in a model called Sonnet, which is the second-largest in their Claude 3 LLM series. They showed that identifiable combinations of multiple neurons, combined in specific proportions, represent human-meaningful concepts in the model's "mind". These features, as the researchers called them, give us a completely new insight into what the LLM is doing, and it's a great sign that they've been found in Sonnet, a 70 billion parameter model. This is still an order of magnitude simpler than today's biggest frontier models, including Anthropic's own Claude 3 Opus, but it's big enough to strongly suggest that the approach can be scaled up further.
Examples of the millions of features found in Sonnet include things like the Golden Gate Bridge, Brain science, and Transit infrastructure, as well as abstractions like Coding error, Lying, and Sycophancy.
Glossing over tremendous amounts of scientific and engineering challenge, what the team did was train another type of neural network model (a "sparse autoencoder", if you care) on a large body of Sonnet's internal states, having it isolate neuron groups whose combined values varied as independently (from each other) as possible. These they took to be the features. But initially, the second model was just as inscrutable as the first! So then they had to explore the discovered features to find out what human concepts they lined up with, doing things like prompting Sonnet with text about the Golden Gate Bridge (or whatever) to see what lit up in the second model's innards. Lo and behold, on testing, they found that features discovered this way were specific and stable in their representation of the associated concepts, and could even be manipulated to cause the LLM to obsess over or ignore specific "ideas".
There's much more to say about this. Just one example: the Anthropic team found that concepts that seem related to us also seem related in Sonnet's "mind":
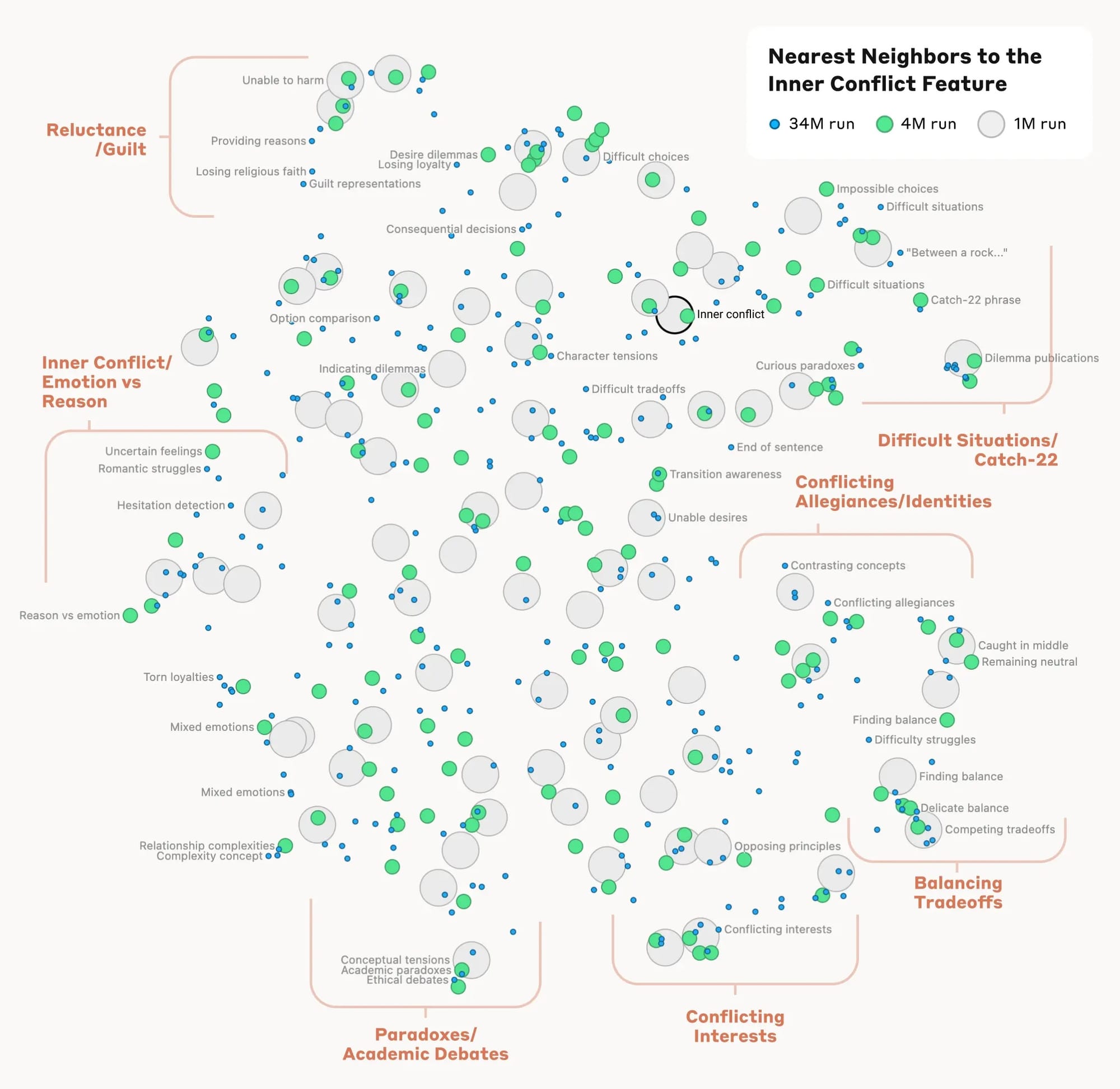
If you'd like to explore further, see Anthropic's own blog post. The general-audience press has also covered this research quite well; for example, Kevin Roose in The New York Times (unlocked link) and Steven Levy in Wired (metered paywall) have interesting comments. The research paper itself has even a great deal more super-interesting depth, and is pretty accessible. (Most readers will need to skim over the technical parts, but won't lose much by doing so.)
It's important to be clear — and the Anthropic team is — that this research in no way means we've "cracked the code" of how the LLM's mind works. Seeing "something about lying" flick by during an experiment doesn't tell you whether the model is planning to lie, or recognizing a lie, or ruling out a response because it would be a lie, or wondering whether its prompter wants it to lie, or having an idle thought about lying that isn't going to affect its final response at all. It doesn't directly tell you where the same feature would come into play outside the experimental setting. It certainly doesn't tell you everything about how the feature you're looking at interacts with millions of other identified features, almost none of which have known correlations with human-recognizable concepts. It's very analogous to the way we've recently learned how to correlate fMRI imaging results with some conscious thought patterns: nobody thinks this means, "Oh, now we know how the human mind works."
Awesome nevertheless!
Quantization
This is what I referred to above as "my personal poster child for how much low-hanging fruit there may still be in AI efficiency improvements". This one is technical, but it's kind of simple.
I'm going to present it as if it were stupid simple, though of course in the real world, there are all sorts of complications and variations, and it takes a lot of intense science and engineering to make this work. But let's ignore that reality and have some fun.
In the beginning, transformers (like what are now used in most LLMs) used standard 32-bit floating point numbers. Such numbers, when converted for human consumption, look something like this:
-2.241776e13
(The part at the end, starting with the letter "e", is an exponent, meaning that our sample number here would be multiplied by 10 raised to the 13th power.)
This numerical format is used all over the place in computing; almost everywhere that doesn't require especially high precision. (Those applications use similar but larger formats that can accommodate more digits.) It follows a standard so stable that it's barely been touched since 2008.
Such numbers, when used in large quantities the way LLMs do, take up a lot of memory, and the computer chips that operate on them are complex. So, moving backwards compared to just about every other application area, the designers of LLMs started to wonder, "What if we tried less precision?"
And it turned out 16-bit numbers worked pretty well! A lot of memory, compute and energy savings for a very modest loss in performance.
So they tried 8-bit numbers. Now this is starting to sound pretty crazy to an old-school programmer like me. In 8-bit floating point, there are only a couple hundred possible numbers. You can't even have the number 17 in 8-bit floating point — that's asking for too much precision; can I interest you in 16 or 18? You can't have anything bigger than 240 — that's as high as it goes! If you want, you can check out the whole space in a table right here.
But guess what? 8-bit quantized models work, and they work pretty well. People have gone on to try 4-bit, and 2-bit, and 1.58 bit (numbers are 1, 0, or -1), and yep, there are now several 1-bit quantizations, including one called BitNet, from Microsoft Research, the University of Chinese Academy of Sciences, and Tsinghua University, that has people pretty excited.
In an LLM quantized using BitNet, every weight in the wall of numbers is either:
+1
or:
-1
This breaks every intuition in my body, but the authors demonstrate that their approach can retain a lot of the performance of bigger models, while cutting energy consumption on the most important metrics by anywhere from one to several orders of magnitude!
If you want more to read...
This is where I usually give you a few links to additional recent topics of interest, but the whole post was such stuff this time, so let me talk about something a little different.
I have a set of pages where I keep links I want to hold on to, sort of a bookmarks-on-steroids system. It's not just for tech, but as you can imagine, in my universe tech in general and AI in particular are well represented.
I've salted away 108 links so far in 2024. A disproportionate number of those — 15 — are from a single source: WIRED magazine. If you're not familiar with WIRED, you might want to consider giving it a look. It's not free, but it's reasonable, currently at $30 per year, discounted to a few bucks for the first year.
I usually access it from their daily newsletter rather than their home page, which I find pretty noisy.
Here's how Wikipedia describes WIRED:
Wired (stylized in all caps) is a monthly American magazine, published in print and online editions, that focuses on how emerging technologies affect culture, the economy, and politics.
In other words, it's for mortals. A few examples of things they've brought me this year that added unique value over and above the copious tech reading I do elsewhere:
- Get Ready for the Great AI Disappointment, but also
- It’s Time to Believe the AI Hype.
- Why the Voices of Black Twitter Were Worth Saving, the latest of Jason Parham's essential articles on Black Twitter. In addition to recognizing and celebrating Twitter's Black voices, Parham is illuminating Twitter's actual unique contribution to our society: providing not only a place for marginalized communities and smaller communities to nurture new public voices, but also a place for those voices — and their ideas (eg #OscarsSoWhite, eg #BlackLivesMatter) — to cross over. This is something that seems entirely lost on most of the press, with many — in premature anticipation of Twitter’s demise — eagerly awaiting the disappearance of its dirty bathwater with no thought at all for the baby.
- 8 Google Employees Invented Modern AI. Here’s the Inside Story.
- You Think You Know How Misinformation Spreads? Welcome to the Hellhole of Programatic Advertising. Read it if you dare.
For the record, I have no relationship with WIRED, and there's never been (and probably never will be) an affiliate link in AI for Mortals. I just thought you might want to check it out.
This article originally appeared in AI for Mortals under a Creative Commons BY-ND license. Some rights reserved.